We are delighted to have these distinguished plenary speakers.
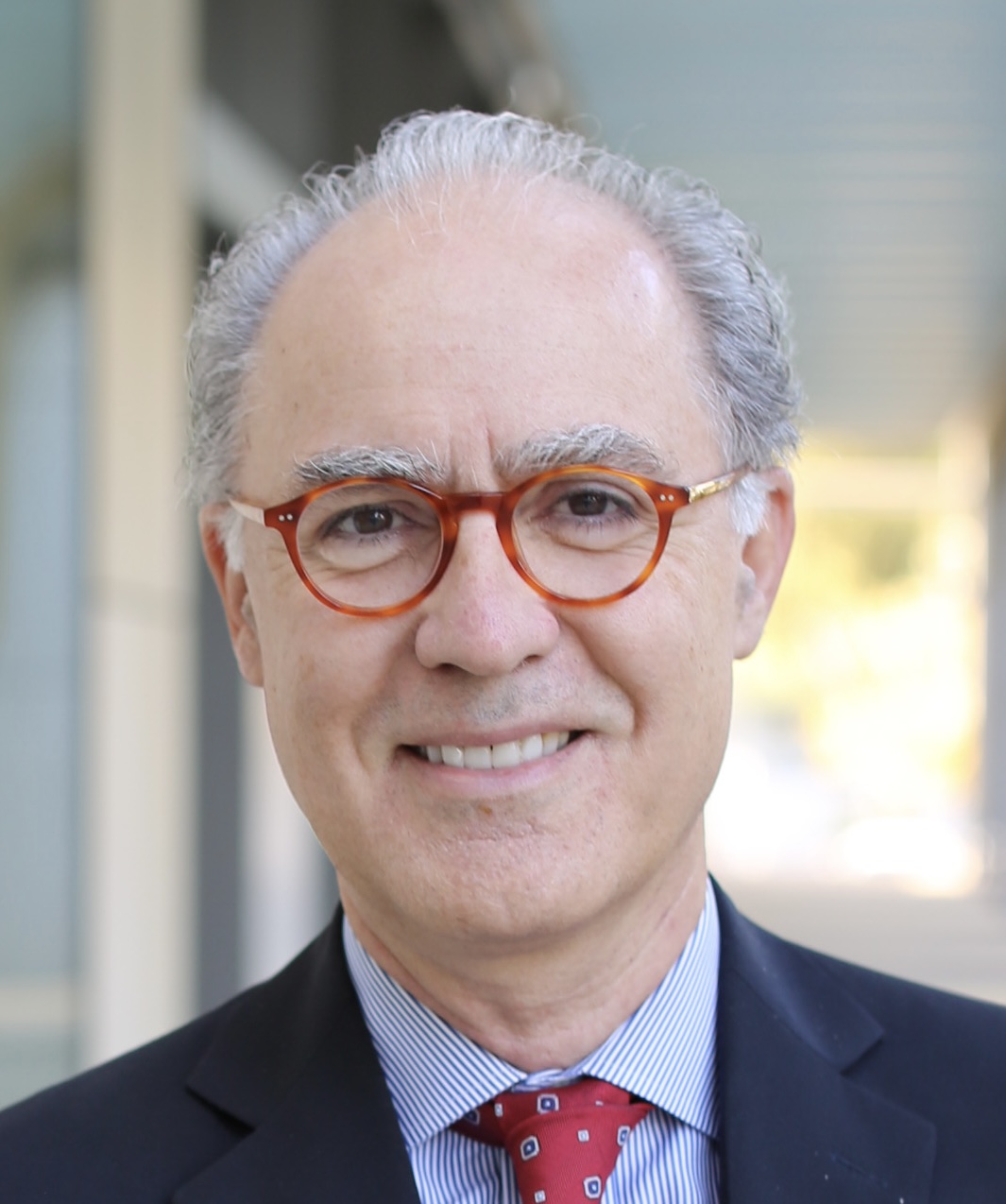
Miroslav Krstic
Senior Associate Vice Chancellor for Research
University of California San Diego
Control and Machine Learning: from Rivals to Partners
Thursday, February 1st, 2024 at 9:15am (Room 32-155)
AbstractIt is no longer a question WHETHER machine learning should be incorporated into the control toolbox but only HOW. Even some high-profile AI pioneers caution about the use of ML for control in a manner where physical modeling - even when possible - is bypassed, and where the distinguishing requirements of feedback systems - stability and safety - are not guaranteed. I propose a use of ML which leverages the control community's heritage of rigorous, certificate-bearing control designs. A game-changing “supporting and empowering role” is entrusted to ML, in automating and accelerating by several orders of magnitude the implementation of model-based control designs. The benefit of such a control+ML blend, where both control and ML get to perform complementary roles for which they are best suited, is nowhere as evident as for hard-to-control systems modeled by partial differential equations. I employ, in PDE control, the recent breakthroughs in deep learning approximations of not functions but function-to-function mappings (nonlinear operators) - the so-called “neural operators.” With neural operators, entire PDE control methodologies are encoded into what amounts to a function evaluation, leading to a thousandfold speedup in real-time implementation, while retaining the stability guarantees. The talk presents joint work with Luke Bhan, Yuanyuan Shi, Maxence Lamarque, and others. Applications range from traffic and epidemiology to manufacturing, energy generation, and supply chains.
BiographyMiroslav Krstic is Distinguished Professor and Senior Associate Vice Chancellor for Research at University of California, San Diego. He has received the IEEE Hendrik Bode Lecture Prize, Richard Bellman Control Heritage Award, SIAM Reid Prize, ASME Rufus Oldenburger Medal, IFAC TC awards for Nonlinear Control, Distributed Parameter Systems, and Adaptive Systems, IFAC’s Harold Chestnut Textbook Prize, the Ragazzini Education Award, the inaugural AV Balakrishnan Award for the Mathematics of Systems, and other recognitions. He has been elected Fellow of seven scientific societies and member of the Serbian Academy of Sciences. Krstic serves as EiC of Systems & Control Letters (currently), EiC-Designate for IEEE Transactions on Automatic Control (2026), and Senior Editor in Automatica. A co-author of 18 books and 450 journal papers, Krstic is one of the high-impact researchers in the field of control systems (h=124).
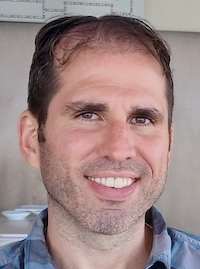
Mark M. Wilde
Associate Professor of Electrical and Computer Engineering
Cornell University
Exact solutions for the fundamental limits of communication, discrimination, and estimation of bosonic dephasing channels
Thursday, February 1st, 2024 at 2pm (Room 32-155)
AbstractDephasing is a prominent noise mechanism that afflicts quantum information carriers, and it is one of the main challenges towards realizing useful quantum computation, communication, and sensing. In the case of bosonic systems, central to many applications, bosonic dephasing channels (BDCs) form a key class of non-Gaussian channels modeling noise affecting superconducting circuits or fiber-optic communication channels. Here we consider communication, discrimination, and estimation of BDCs, when using general strategies for these tasks as allowed by quantum mechanics. We provide an exact formula for the quantum, private, two-way assisted quantum, and secret-key agreement capacities of all BDCs, proving that that they are all equal to the relative entropy of the distribution underlying the channel to the uniform distribution. For discrimination and estimation tasks, we reduce difficult quantum problems to simple classical ones based on the probability densities defining the BDCs. We present upper bounds on the performance of various distinguishability and estimation tasks and show that they are also achievable. To the best of our knowledge, this is the first example of a non-Gaussian bosonic channel for which there are exact solutions for all of these tasks. Joint work with Zixin Huang (Macquarie University) and Ludovico Lami (University of Amsterdam).
BiographyMark M. Wilde received the Ph.D. degree in electrical engineering from the University of Southern California, Los Angeles, California. He is an Associate Professor of Electrical and Computer Engineering at Cornell University. He is an IEEE Fellow, he is a recipient of the National Science Foundation Career Development Award, he is a co-recipient of the 2018 AHP-Birkhauser Prize, awarded to “the most remarkable contribution” published in the journal Annales Henri Poincare, and he is an Outstanding Referee of the American Physical Society. His current research interests are in quantum Shannon theory, quantum computation, quantum optical communication, quantum computational complexity theory, and quantum error correction.
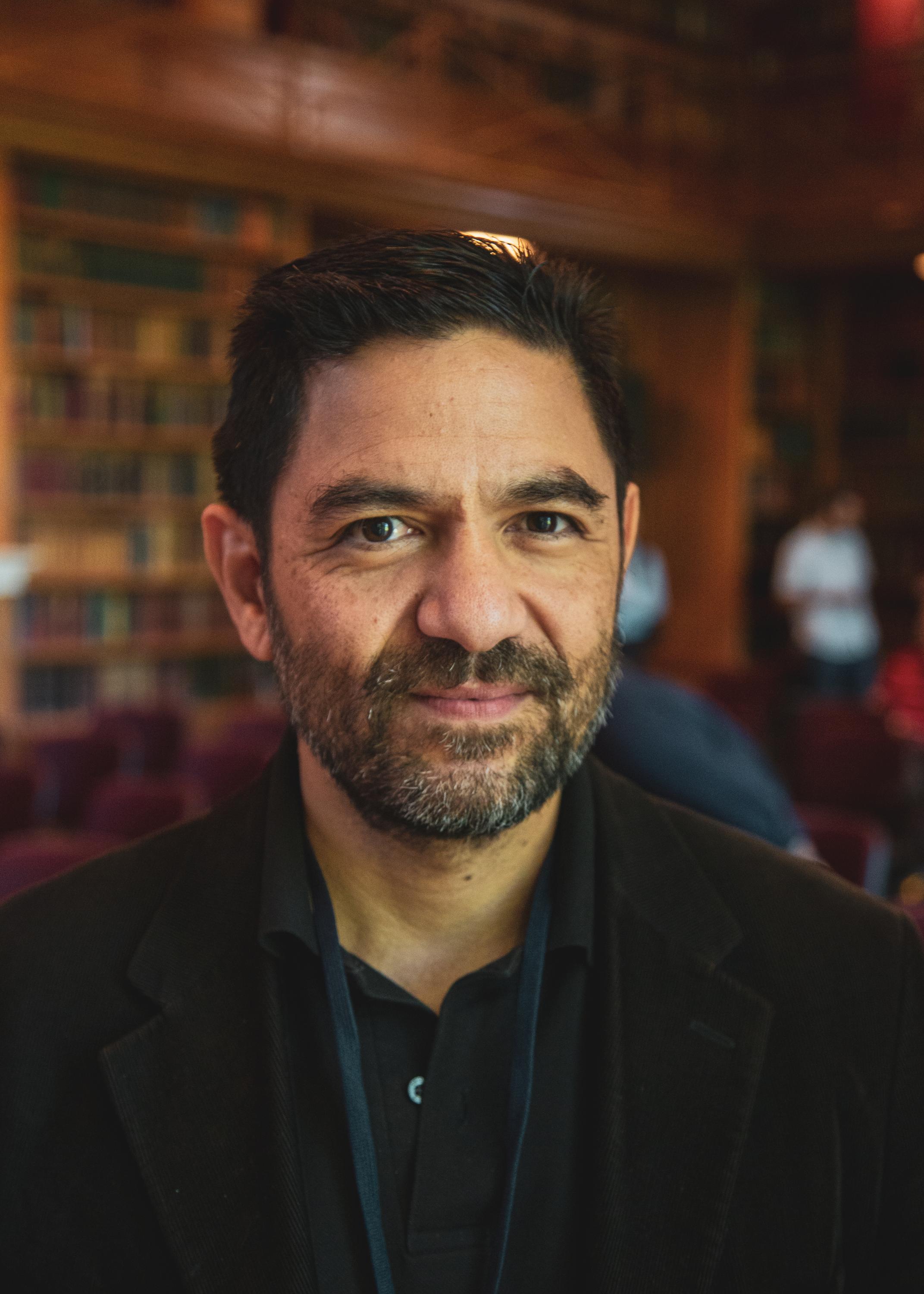
Benjamin Van Roy
Professor of Electrical Engineering
Stanford University
Exploration at Scale
Friday, February 2nd, 2024 at 9am (Room 32-155)
AbstractIn producing today’s generative AIs, interactive learning primarily serves to filter what is learned from massive data corpi, encouraging responses that imitate more desirable content. Though AIs are pretrained on trillions of data bytes, even a hundred thousand bits of human feedback greatly improves behavior. And this in spite of the interactive data being gathered without the benefit of sophisticated exploration algorithms. It is conceivable that more efficient exploration coupled with the rapidly growing volume of human interaction will enable superhuman creativity. I will discuss recent results from applying uncertainty estimation and exploration algorithms in training generative AIs, as well as foundational work that supports these methodologies.
BiographyBenjamin Van Roy is a Professor of Electrical Engineering and Management Science and Engineering at Stanford University. He also leads a research team at Google DeepMind. His research interests center on the design of reinforcement learning algorithms that expand artificial intelligence capabilities. He received his SB, SM and PhD from MIT, where his doctoral research was advised by John N. Tsitsiklis. While at MIT, he received the George C. Newton Undergraduate Laboratory Project Award, the Morris J. Levin Memorial Master's Thesis Award, and the George M. Sprowls Doctoral Dissertation Award. He is a Fellow of IEEE and INFORMS and a recipient of the INFORMS Frederick W. Lanchester Prize.
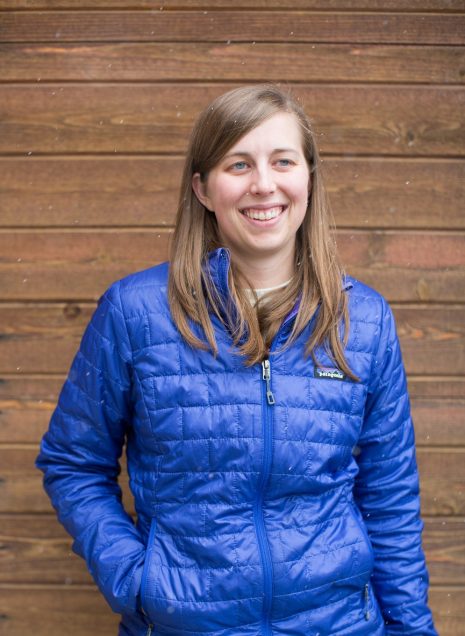
Alyssa Pierson
Assistant Professor of Mechanical Engineering
Boston University
Improving Interactions Among Semi-Cooperative Robots
Friday, February 2nd, 2024 at 2pm (Room 32-155)
AbstractRobots will transform our everyday lives, from home service and personal mobility to large-scale warehouse management and wildlife monitoring. Across these applications, robots need to interact with humans and other robots in complex, dynamic environments. Understanding how robots interact allows us to design safer and more robust systems. This talk presents an overview on how we can integrate underlying cooperation and interaction models into the design of the robot teams. We use tools from behavioral decision theory to design interaction models, combined with game theory and control theory to develop distributed control strategies with provable performance guarantees. This talk focuses on teams of robots interacting with cluttered environments, with applications in autonomous driving, resource delivery, and wildlife monitoring.
BiographyAlyssa Pierson is an Assistant Professor of Mechanical Engineering at Boston University. Her research interests include trust and cooperation in multi- agent systems, distributed robotics control, and socially-compliant autonomous system design. She focuses on designing robotic systems that interact with humans and other robots in complex, dynamic environments. Prior to joining BU, Professor Pierson was a research scientist with the Computer Science and Artificial Intelligence Laboratory (CSAIL) at MIT. She received her PhD degree from Boston University in 2017 and BS in Engineering from Harvey Mudd College. During her PhD, she was awarded the Clare Booth Luce Fellowship. She was a Best Paper Finalist at the 2016 International Conference on Robotics and Automation, an Honorable Mention for the 2022 IEEE Transactions on Robotics King-Sun Fu Memorial Award, a recipient of the NSF CAREER award in 2023, and received the inaugural MassRobotics Rising Star Award in 2023.