Plenary speakers
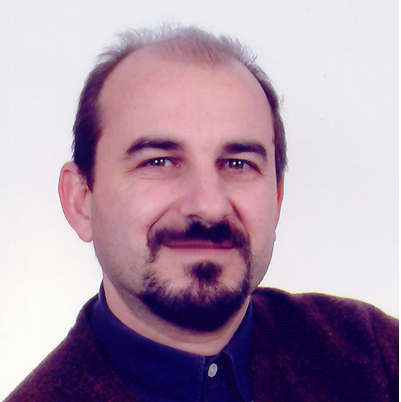
Marco Chiani
Professor
University of Bologna
Recent Advances in Quantum Surface Codes: Analysis, Design, and Decoding
AbstractClassical error-correcting codes are essential for protecting information from noise and interference, playing a critical role in communication and computing systems. Common classical noise models include bit-flips (e.g., "00101100" ⇒ "01101100"), bit-erasures (e.g., "00101100" ⇒ "0?101100"), and Additive White Gaussian Noise (AWGN). In quantum computing and communication, the mitigation of noise is even more crucial due to inevitable interactions with the environment and the inherent challenges in processing quantum information. This talk introduces quantum error correction and presents recent advances in the development of quantum surface codes. It emphasizes the key differences between classical and quantum error correction and provides an overview of the current progress in the analysis, design, and decoding of these codes.
BiographyMarco Chiani is a Professor of Telecommunications at the University of Bologna. He received the Dr. Ing. degree in Electronic Engineering and the Ph.D. degree in Electronic and Computer Engineering from the University of Bologna, Italy, in 1989 and 1993, respectively. Since 2003, he has been a frequent visitor to the Massachusetts Institute of Technology, as a Research Affiliate. He is an IEEE Fellow and has been appointed a Distinguished Visiting Fellow of the Royal Academy of Engineering, U.K. He has received several awards, including the 2011 IEEE Abraham Prize, the 2012 IEEE Ellersick Prize, and the 2012 IEEE Rice Prize in the field of Communications Theory. He also served as the Chair (2002–2004) of the Radio Communications Committee of the IEEE Communications Society. His research interests span information theory, wireless systems, statistical signal processing, and quantum information. His contributions include exponential bounds for the Gaussian error function and the statistical distribution of the eigenvalues of random matrices.
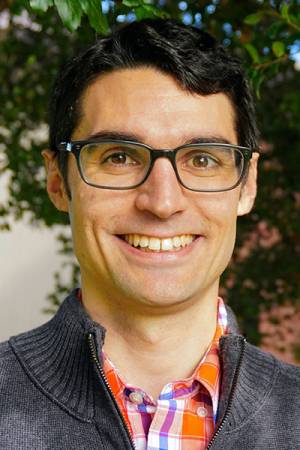
Zac Manchester
Assistant Professor
Carnegie Mellon University
Numerical Methods for Things That Move
AbstractRecent advances in motion planning and control have led to dramatic successes like SpaceX’s autonomous rocket landings and Boston Dynamics’ humanoid robot acrobatics. However, the underlying numerical methods used in these applications are typically decades old, are not tuned for high performance on control problems, and are often unable to cope with the types of optimization problems that arise naturally in modern robotics applications like legged locomotion and autonomous driving. This talk will focus on numerical optimization tools built explicitly to solve such challenging motion planning and control problems at real-time rates to enable robotic systems that move with the same agility, efficiency, and safety as humans and animals. I’ll also highlight recent applications including humanoid robots, quantum computers, and satellite swarms.
BiographyZac Manchester is an Assistant Professor of Robotics at Carnegie Mellon University. He holds a Ph.D. in aerospace engineering and a B.S. in applied physics from Cornell University. Zac was a postdoc in the Agile Robotics Lab at Harvard University and has previously worked at Stanford, NASA Ames Research Center, and Analytical Graphics, Inc. He received a NASA Early Career Faculty Award in 2018 and a Google Faculty Research Award in 2018, and he has led four NASA-funded satellite missions. His research interests include motion planning, control, and numerical optimization, particularly with application to robotic locomotion and spacecraft guidance, navigation, and control.
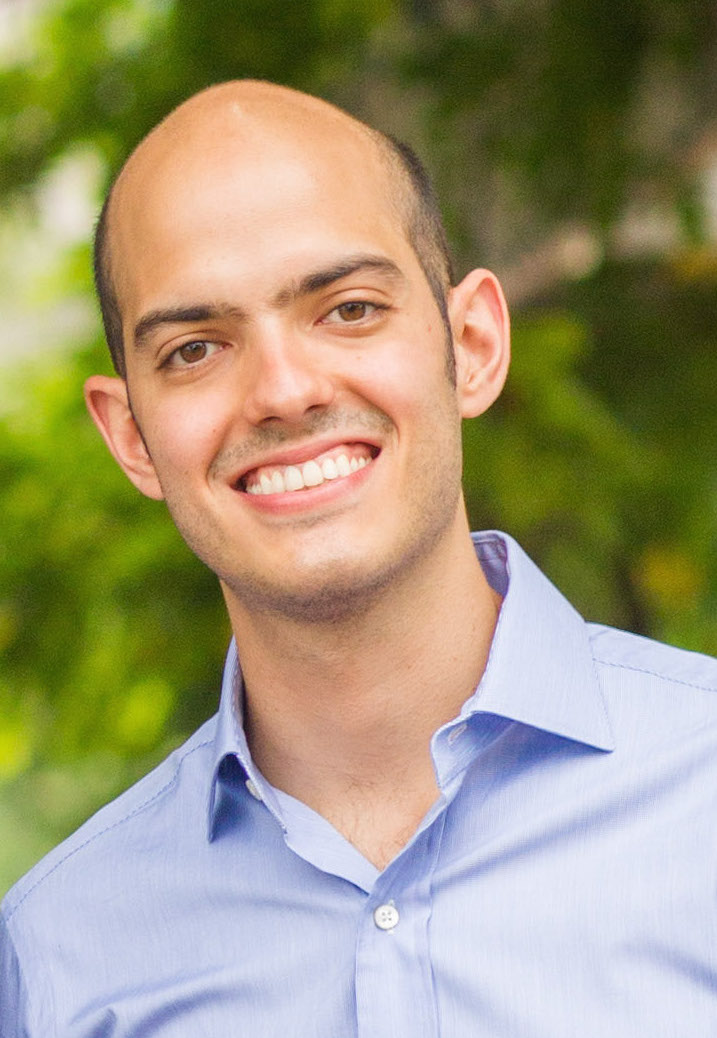
Flavio du Pin Calmon
Associate Professor
Harvard University
A World of Divergences
AbstractThis talk overviews information-theoretic results on trustworthy machine learning and artificial intelligence. We first highlight recent progress at the interface of information theory and differential privacy, including new methods for privacy accounting and the design of privacy mechanisms with favorable privacy-utility trade-off in the large composition regime. We then discuss achievability and converse results for group fairness in prediction and classification tasks. Finally, we overview recent methods for measuring and promoting multi-group proportional representation in image retrieval and generation tasks.
BiographyFlavio P. Calmon is an Associate Professor of Electrical Engineering at the Harvard John A. Paulson School of Engineering and Applied Sciences. Before joining Harvard, he was the inaugural Data Science for Social Good Post-Doctoral Fellow at IBM Research in Yorktown Heights, New York. He received his Ph.D. in Electrical Engineering and Computer Science at MIT. His research develops information-theoretic tools for trustworthy and reliable machine learning.
Prof. Calmon has received the 2024 James L. Massey Award from the IEEE Information Theory Society, the NSF CAREER award, faculty awards from Google, IBM, and Amazon, and the Harvard Dean of Undergraduate Studies Commendation for “Extraordinary Teaching during Extraordinary Times.” He also received the inaugural “Título de Honra ao Mérito” (Honor to the Merit Title) given to alumni from the Universidade de Brasília (Brazil).
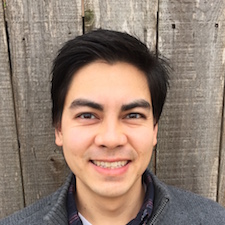
Alex D'Amour
Staff Research Scientist
Google DeepMind
Thinking about Reliability and Trustworthiness in a New Paradigm of Machine Learning
AbstractIn a talk that poses more questions than it answers, I will discuss how I have seen the landscape of reliability and trustworthiness in machine learning change over the last few years. I will provide some of my own views about interesting directions, and some thoughts on navigating a changing research environment.
BiographyAlexander D'Amour is a Staff Research Scientist at Google DeepMind, based in Cambridge, MA. Alex's work focuses on improving the reliability, fairness, and robustness of ML/AI systems, often using the formalism of causality. Prior to Google, Alex received his PhD in Statistics at Harvard University, and was a Visiting Assistant Professor at the UC Berkeley Department of Statistics. Alex has worked in a number of application areas, including healthcare, sports analytics, healthcare, and education.